Solving Shelf-Share, Purity and Planogram Compliance in retail execution using Artificial Intelligence
At SharedReach Mobility Services Pvt. Ltd
(2018-2020)
AI-based research project to identify the presence of products in retail shelf images and evaluate their share on shelves & their adherence to the given planogram. The objective of the project was to develop a solution for SharedReach to automate the manual auditing of images using computer vision and deep learning techniques.
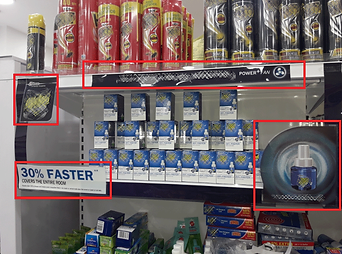
Element presence and planogram solved by deep neural networks
One of the core features of the SharedReach platform "Ubora" is to provide its client with detailed insights into their execution in the market. SharedReach employs a fleet of field teams across the country, who visit outlets of the brands on a planned basis and report visuals & data using the Ubora mobile app. At the backend, a team of auditors manually evaluate this data to generate reports, scorecards, identify gaps in the execution, determine shelf share, planogram compliance, etc hence providing brands detailed information about their execution.
​
This research project aimed to automate and simplify the task of manual auditing using computer vision and artificial intelligence. With enough historical data accumulated over time, we conducted a complete AI development cycle from labeling images to modeling, training, evaluating various neural networks to fine-tune parameters and obtain accuracy. We conducted pilots and developed many prototypes for clients dealing with different kinds of real-life challenges and conditions to provide the following solutions:
Planogram Compliance
Identify various elements such as objects, SKUs, POSMs, creatives, etc in the images and evaluate their adherence with a given planogram for in-store execution.
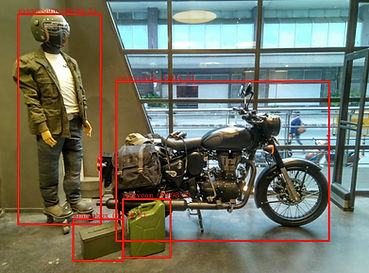
Planogram compliance using AI in showrooms.

Planogram compliance using AI in general trade outlets.
Shelf-Share and Purity
Identify elements in the shelf images and evaluate various parameters such as:
-
Total expected products that can fit on the shelf.
-
Observed product count: No of products on each shelf (only facings).
-
Brand's products count: No of products of a particular brand seen on the shelf.
-
Fill Rate: Percentage occupancy of the shelf which is the ratio of 'Observed product count' vs 'Total expected products'.
-
Purity: Percentage of 'brand products' present from the 'observed products'.
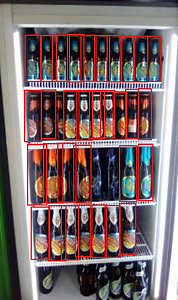
Fill rate and purity analysis using AI in visicoolers.
Total expected #:
44
Observed product #:
38
Brand's products #:
32
Fill Rate:
86.36 %
Purity:
84.21%
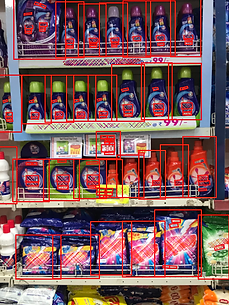
Shelf share analysis using AI in retail execution.
Observed product #:
30
Brand's products #:
27
Fill Rate:
90.00 %
My Role
As an employee of SharedReach, I led this project as an Applied AI/ML Engineer. I learned computer vision and artificial intelligence for more than 4 years with my mentor and together, we applied our learnings to create a solution for the company.
With significant success, we created a team of 5-6 people which included Data Scientists, Business Analysts, Annotation Executives led by me and directed by the Director of the company.
​
The project received great appreciation from the management & clients and it eventually became one of the hot-selling and innovative products of the company.
SharedReach Mobility Services Pvt. Ltd.
Gurugram, India
DISCLAIMER: All rights reserved. All images, logos, content, etc are trademarks of SharedReach Mobility Services Pvt. Ltd. and may be legally privileged. The sole purpose of this page is to present my role and contribution to the project.